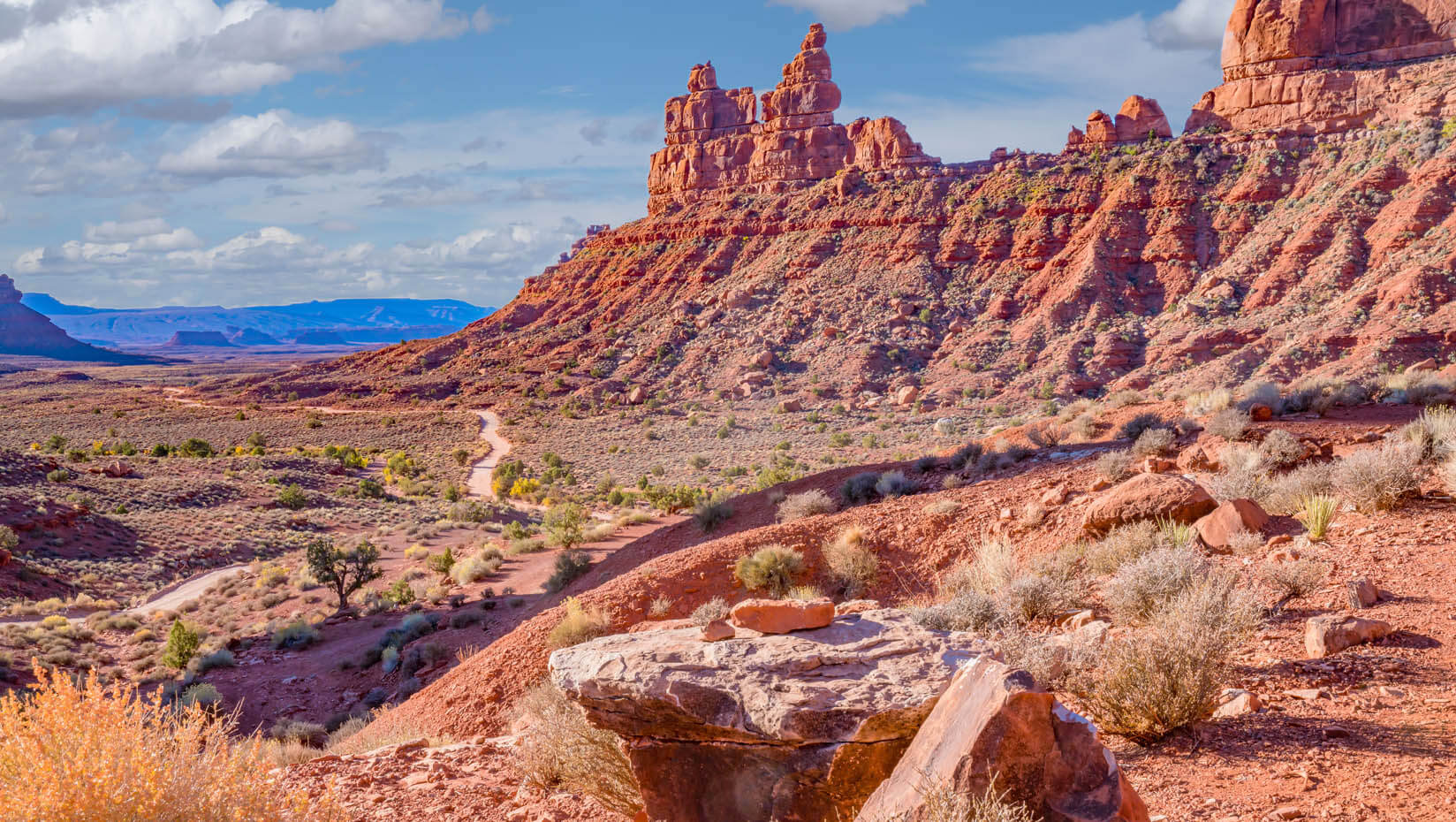
UMaine researcher’s study reveals public sentiment toward National Monument review using AI
Public comments can help government officials evaluate potential policy decisions that affect national monuments and other federal land. The introduction of online comments, however, has brought staggering amounts of feedback that can be difficult to summarize, and can bury concerns federal agencies should consider.
Caitlin McDonough MacKenzie, a postdoctoral research fellow with the University of Maine Climate Change Institute, led a team of postdoctoral conservation researchers in testing the use of a machine learning algorithm to quantify public sentiment toward decisions involving federal land. They reported their findings in a study published in the journal Conservation letters.
“AI (artificial intelligence) like our machine learning program empowers conservation biologists with the ability to focus on the trends and patterns rather than the raw data,” says Tony Chang, study co-author and data scientist at Conservation Science Partners.
The group tasked a deep recurrent neural network with analyzing more than 750,000 remarks submitted during the 2017 public comment period for the Department of the Interior’s executive review of 27 national monuments. The review resulted in the federal government reducing the footprints of the Bears Ears and Grand Escalante national monuments in Utah.
The Interior Department at the time dismissed comments that were critical of the review as “a well‐orchestrated national campaign organized by multiple groups.” McDonough MacKenzie and her colleagues, however, found using machine learning that out of the comments submitted by individuals, not organizations or bots that would typically be used in campaigns, 97.4% expressed opposition toward the review. The finding suggests overwhelming support for maintaining national monument designations, says McDonogh MacKenzie, also a visiting assistant professor at Colby College.
“We started this project as a group of conservation postdocs. We wrote one of the 750,000 public comments in response to the review of National Monuments. When the Trump administration dismissed our perspective, we started wondering ‘What was the real public sentiment toward National Monuments — not in form letters, but in individual comments? And did this align with our letter and our work in conservation?’” she says. “This research actually says we’re in good company — 97.4% of humans writing original comments opposed this review.”
Colleagues from the David H. Smith Conservation Research Fellows worked on the project with McDonough MacKenzie. Participants included Michael Dombeck, executive director of the fellowship, former acting director of the Bureau of Land Management and former U.S. Forest Service chief under President Bill Clinton.
The team had to teach their algorithm to differentiate between comments with positive language about the national monuments themselves and responses with positive language expressed toward the review of said monuments. McDonough MacKenzie said the task proved to be the hardest during the project.
During their analysis, McDonough MacKenzie and her colleagues were eliminating duplicate comments from bots in the Interior Department’s review, and they discovered the repeat feedback overshadowed individual comments and form letters. In response to their discovery, researchers tasked their AI with grouping all comments based on whether they derived from humans, organizations or bots.
The deep recurrent neural network found that out of the more than 750,000 comments submitted in response to the monument review, 20% derived from human individuals, 11% came as form letters, or “individual comment(s) drafted by nongovernmental organizations and customized for submission by humans,” and 69% originated from bots, according to researchers.
Human comments were defined and identified based on their complete uniqueness from other comments. The AI discovered form letter comments by pinpointing collections of very similar comments that contained small differences from one another, typically in the form of a submitter’s name or a custom sentence. According to researchers, comments from bots were detected when the AI identified complete duplications of text, although some contained combinations of text from different form letters that were submitted tens of thousands of times.
The AI also found that 96.4% of form letter comments and 99.6% of bot comments expressed opposition toward the Interior Department’s national monument review.
“Through machine learning, we discovered that it’s not form letter campaigns that are overshadowing individual public comments, but bots,” McDonough MacKenzie says. “In this case, excluding form letters and bots does not change public sentiment, because unique, individual comments resoundingly opposed the national monuments review. But across all public comments, bots hamper public participation in comment periods — they reduce the impact of individuals and drown out the best available science.”
McDonough MacKenize says automated software bots that mimic human participation in online forums can disrupt the public comment process through manipulation, and the Interior Department should have addressed the number of bot comments submitted for the review in its reporting. The lack of captcha security in online feedback submissions and insufficient monitoring for bot comments raises concerns about policy decision making for public land, she says.
“AI is not science fiction anymore. It’s a real tool we are using to tackle problems that used to be dismissed for their vastness,” says Mallika Nocco, study co-author and an assistant Cooperative Extension specialist in soil-plant-water relations and irrigation management with the University of California-Davis Department of Land, Air and Water Resources. “No more. We can now compel and expect our government to hire conservation scientists, like us, to listen and respond to the public when they take the time to write a comment.”
Contact: Marcus Wolf, 207.581.3721; marcus.wolf@maine.edu