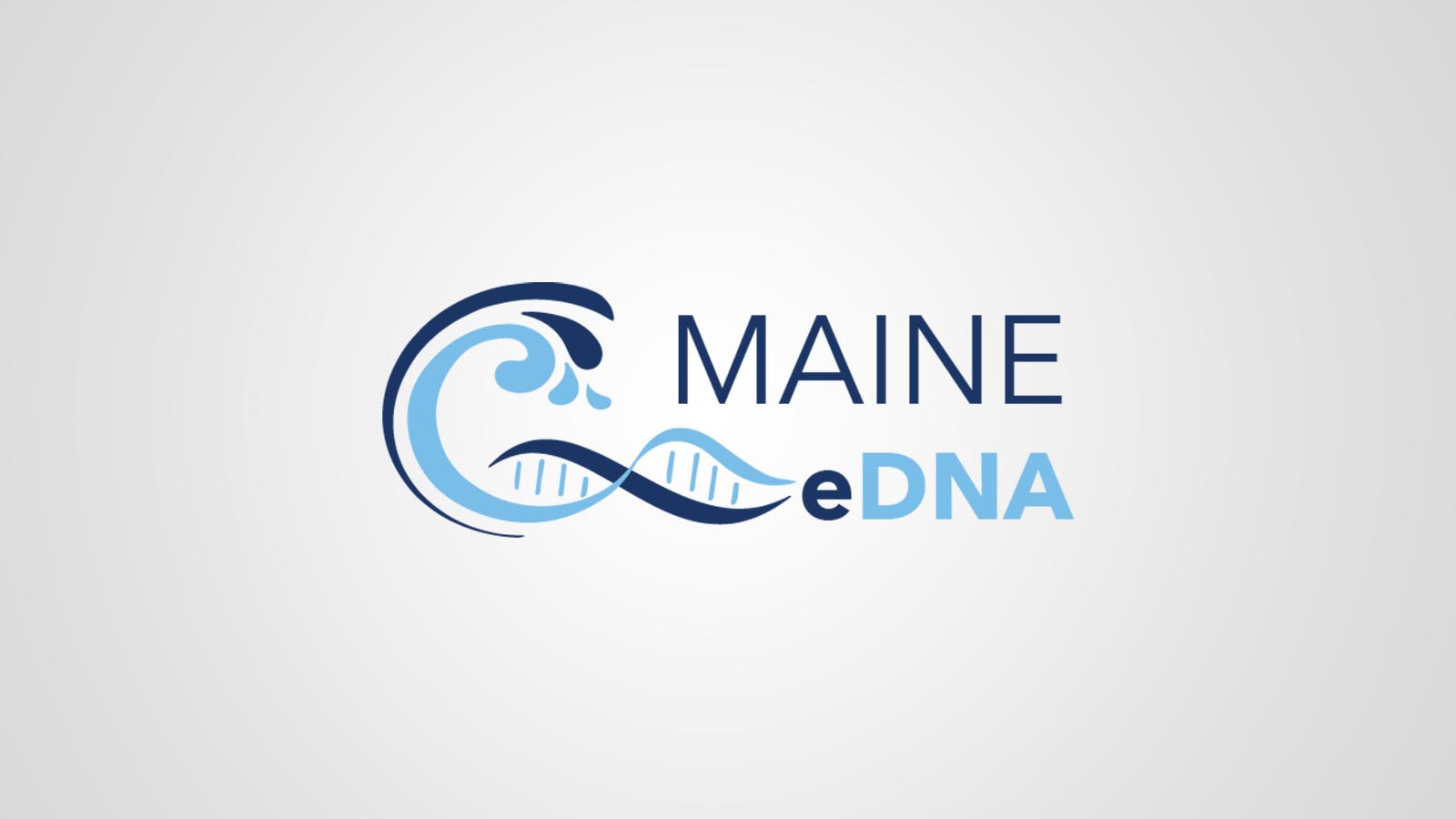
New Faculty Hire: Chaofan Chen
By Attis Bielecki, ME EPSCoR Student Writer
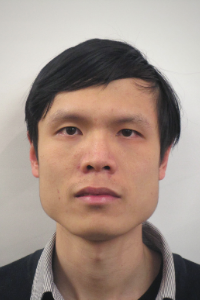
Dr. Chaofan Chen is an Assistant Professor at the University of Maine in the School of Computing and Information Science. He joined the National Science Foundation EPSCoR RII Track-1 award, Maine-eDNA, in September 2020. With his Ph.D. in computer science from Duke University, he is guiding data science activities on the Maine-eDNA program. He also finds himself rapidly learning about biology and bioinformatics, which he hopes will help him develop a wide array of machine learning tools for analyzing and understanding environmental DNA (eDNA).
“The field of machine learning involves training a model, which is essentially a computer program, to perform a particular task by showing the model lots of examples, so that the model can learn from those examples,” Chen described. “For instance, we could train a model to recognize birds by showing the model lots of pictures of birds, and also telling the model that some of these birds are sparrows and others are seagulls. We will train the model to the point where we can remove the labels and the model is able to properly identify the bird without being told what kind of bird is in the photo.”
The areas of artificial intelligence (AI) and machine learning are very broad, but Chen has concentrated his efforts. “My specific focus is called ‘explainable AI’ or ‘interpretable machine learning,” Chen explained.
The research into the application of machine learning with eDNA analysis is still in the early stages of development. Chen has started by collecting and training machine learning models on publicly available data and will eventually use the data collected by Maine-eDNA researchers. Chen believes that this work will help the Maine-eDNA program gain insight into eDNA and lead to novel AI tools that will help with environmental monitoring.
Chen’s goal is to make models more understandable to humans. For example, instead of simply classifying a photo of a bird as that of a sparrow, the model would also be able to tell people why it classified the photo as a sparrow and not another kind of bird. Chen hopes to apply these human-interpretable machine learning models to analyzing eDNA data. For example, researchers could train a human-interpretable machine learning model to predict if an eDNA sample came from polluted water or clean water. Such a model would not only allow Maine-eDNA personnel to develop an eDNA application that can monitor water quality, but also provide reasons (based on the presence or absence of certain eDNA signals) for why the model predicted that the water is polluted.