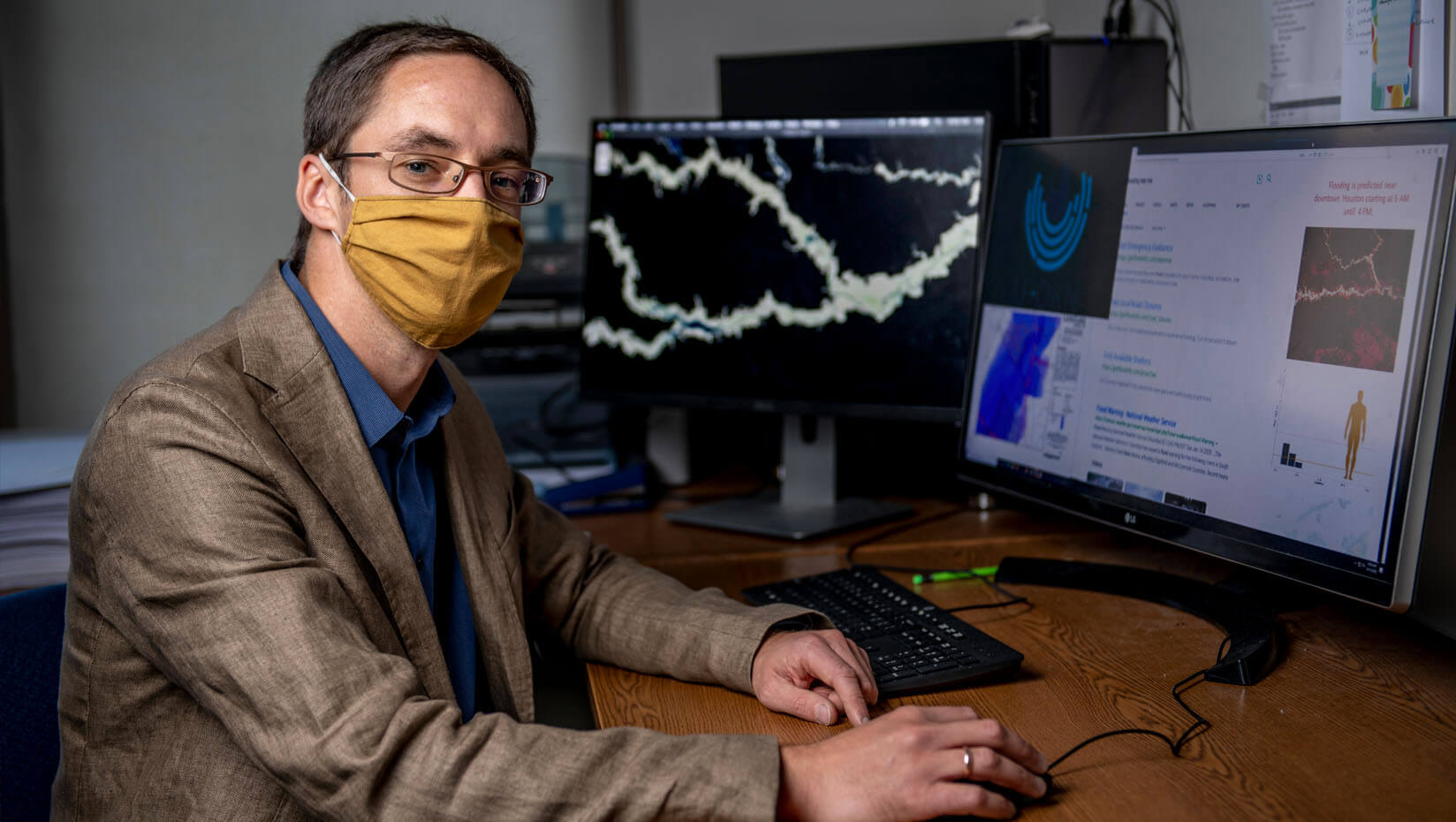
UMaine computer scientist helps develop NSF-backed AI to predict, track flood damage to city infrastructure, services
When a flood strikes a city, the damage can ripple through its many interconnected systems of infrastructure and services. Surging waters inundating a culvert or tunnel can prevent access to a particular neighborhood. If a substation shuts down, a group of homes or a hospital can lose power.
Despite the interwoven nature of infrastructure, no tool can holistically predict or track the ramifications of a flood event in a metropolitan area. A coalition of scientists, including one from the University of Maine, hope to develop a program governed by artificial intelligence that will identify what homes and neighborhoods would be inundated, what roads would be inaccessible, what systems would become inoperable and what areas would need evacuation during a flood.
Torsten Hahmann, an associate professor of spatial informatics at UMaine, teamed up with researchers from across the country to create the Urban Flooding Open Knowledge Network (UF–OKN). The University of Cincinnati is leading the development, which is backed with about $6 million from the National Science Foundation.
The UF–OKN will not only provide hydrological projections for a city facing a flood, but also identify which neighborhoods, businesses, roads, dams and public health, water, sewer and power systems would be threatened. The network will forecast probable courses of a flood event 48 hours before it reaches a city, with predictions updated every half hour. It will also provide real-time, high resolution data that allows emergency managers and other stakeholders to track damage and crises created by a flood, Hahmann says.
“At its core, the UFO-OKN uses a knowledge graph that brings together ontologies and data. The technology of knowledge graphs and ontologies is an important piece of the overall landscape of AI tools,” says Hahmann, also director of the Spatial Knowledge and Artificial Intelligence (SKAI) lab at UMaine. “It provides computers with the human knowledge necessary to correctly interpret and connect vast amounts of disconnected information. It has been evolving for a number of years, but there really hasn’t been a big use case for us to showcase what the technology could do (until now).”
The knowledge network will serve as a customizable database providing users, including homeowners, emergency responders, water and sewer system operators, agencies and more, with the information they need to prepare and maneuver through a flood event.
Hahmann says information from the National Oceanic and Atmospheric Administration, the U.S. Geological Survey, cities, states, power companies and other sources will provide the backbone for network forecasts. Stakeholders will be able to incorporate their own, private data to tune the predictions and real-time information based on public information to their needs.
Users accessing the UF–OKN on their computers or smartphones will see a map visualization of the dependencies between different infrastructure systems in a city, and how the inundation of one could affect another, by linking them to each other like a web. For example, if a bridge that grants access to a neighborhood becomes flooded, network users would see on a map, chart or other visual that the neighborhood would become unreachable, Hahmann says.
The UF–OKN will not only help cities prepare for an imminent flood and respond to it when it strikes, but also provide information to help municipal leaders bolster their communities against future inundation, according to the researchers.
“It’s really one pool of information that feeds multiple purposes and multiple users,” Hahmann says.
Hahmann has been structuring the UF–OKN knowledge graph with colleagues from the project team, particularly focusing on the semantics that will govern it. According to the UMaine professor, the graph in the network semantically describes the data and connects users to diverse, yet related information about a location or topic.
For example, when a user searches “coffee shop near me” on a search engine, the computational semantics of what a coffeeshop is and how people use it provides locations, hours, menus and reviews with coffee shop names, according to Hahmann and his colleagues. Semantics will allow the UF–OKN to describe how infrastructure systems affect each other when flooded in a similar fashion.
Hahmann says he and his team need to develop the UF–OKN to establish the necessary semantic connections that turn the existing data into an AI system. In addition to connecting information from different components of urban infrastructure, the UMaine professor says the program will also help model and explain weak points in the infrastructure and how stakeholder decisions’ during a disaster affect other systems or neighborhoods.
“It’s pointless to find patterns that may or may not make sense, that may be coincidental or have no value if you don’t identify their connections or don’t have the terminology to explain them,” he says.
The NSF awarded about $6 million for the project in two separate allocations, both as part of the organization’s Convergence Accelerator. Hahmann’s work will be financed with $253,500 from the NSF funds.
Shirly Stephen, a Ph.D. student of spatial information science and engineering, helped the UMaine professor gather and encode the relevant hydrological and semantics last year, and he says he hopes to recruit another Ph.D student to assist him for the next two years.
The team began its work in 2019. In addition to fleshing out the knowledge graph and other technological components, Hahmann says the group has been contacting agencies and utilities for data.
Hahmann says the team hopes to present a pilot version of the UF–OKN that will represent a particular city in 2021. By demonstrating the capabilities of the network, he says he hopes to attract more municipalities, including those in Maine, and other potential users to buy into the network to support its ongoing development.
“It’s technology that actually helps improve decision making and saves lives,” Hahmann says. “We’re really trying to address a real problem, a pressing concern that could benefit from this technology.”
Contact: Marcus Wolf, 207.581.3721; marcus.wolf@maine.edu